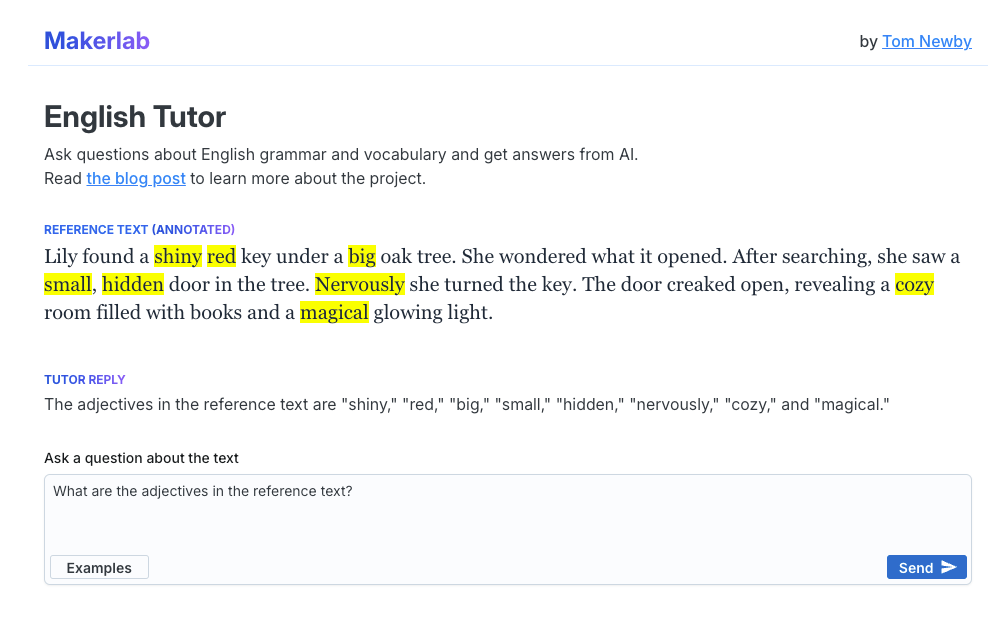
View Demo: English Tutor demo
Brief
I've been experimenting with LLMs lately - finding them to be really fascinating! I gave a talk in July 2024 to BrisPHP developers about LLMs and how they can be used in application development.
What I wanted to experiment with here was using an LLM in a mechanism that is neither a chat interface, nor a basic summarisation task, as we've seen enough of those already.
What I wanted to test the waters on was having the LLM "augment" the UI.
The idea
The idea was to have a reference text (i.e. a few sentences) that the user can ask questions about. Instead of "the system" simply answering the question in a chat thread, "the system" can also highlight parts of the reference text if it thinks it would help communicate.
The build (webapp bits)
This was built on Cloudfront Pages/Page Functions, with a React frontend. The Page Functions invoke Langchain.js callables which are instrumented with Langsmith.
The trickiest bit for me was determining the best way to have the LLM "augment" the UI. I first tried getting the LLM to respond like below:
{
"response": "Lily found a <Highlight>shiny</Highlight> <Highlight>red</Highlight> key under a <Highlight>big</Highlight> oak tree."
}
My thinking here was I have a <Highlight>
React component to handle the highlighting and it should be reasonable to
parse the response and render the highlights. The code I ended up with to parse the response was a bit gnarly and didn't
spark joy.
I then refactored to something more like below, which was felt a lot simpler to implement the React side of things, but required more nudging on the LLM side.
{
"response": [
"Lily found a ",
{
"type": "highlight",
"content": "shiny"
},
" ",
{
"type": "highlight",
"content": "red"
},
" key under a ",
{
"type": "highlight",
"content": "big"
},
" oak tree."
]
}
The LLM bits
I first started trying to get the LLM (OpenAI, various models) to answer all in one go, respond with both the text answer, and the augmented text response as well. What I found was that the LLM struggled with answering the question generally (following the instructions about how to answer) when it was also tasked with generating a structured output for the UI.
As with many things with LLMs, I found that breaking the problem down into smaller, more manageable pieces was the way to go. I ended up with a chain of Langchain callables:
- Answer the question (without knowing about the UI)
- Plan how the UI should be augmented
- Generate the response data following the annotation plan
Model selection
There was enough signs that I could get gpt-4o-mini
to work for this task, but I ended up just using
gpt-4o-2024-08-06
as it was more correct with less prompting work. I setup a simple Langsmith experiment (with only
a few examples) to evaluate the two models.
If I were to continue with this, I would likely setup some more benchmarks in a Langsmith experiment (not sure how evals would work here)
to work on prompting to get gpt-4o-mini
to work better at a lower cost.
My gut is that few-shotting a few examples would quickly nudge the model in the right direction.